Transform Workforces with Predictive AI Data Analysis
Transform Workforces with Predictive AI Data Analysis
How predictive analysis delivers actionable labor market insights and data-driven workforce planning strategies
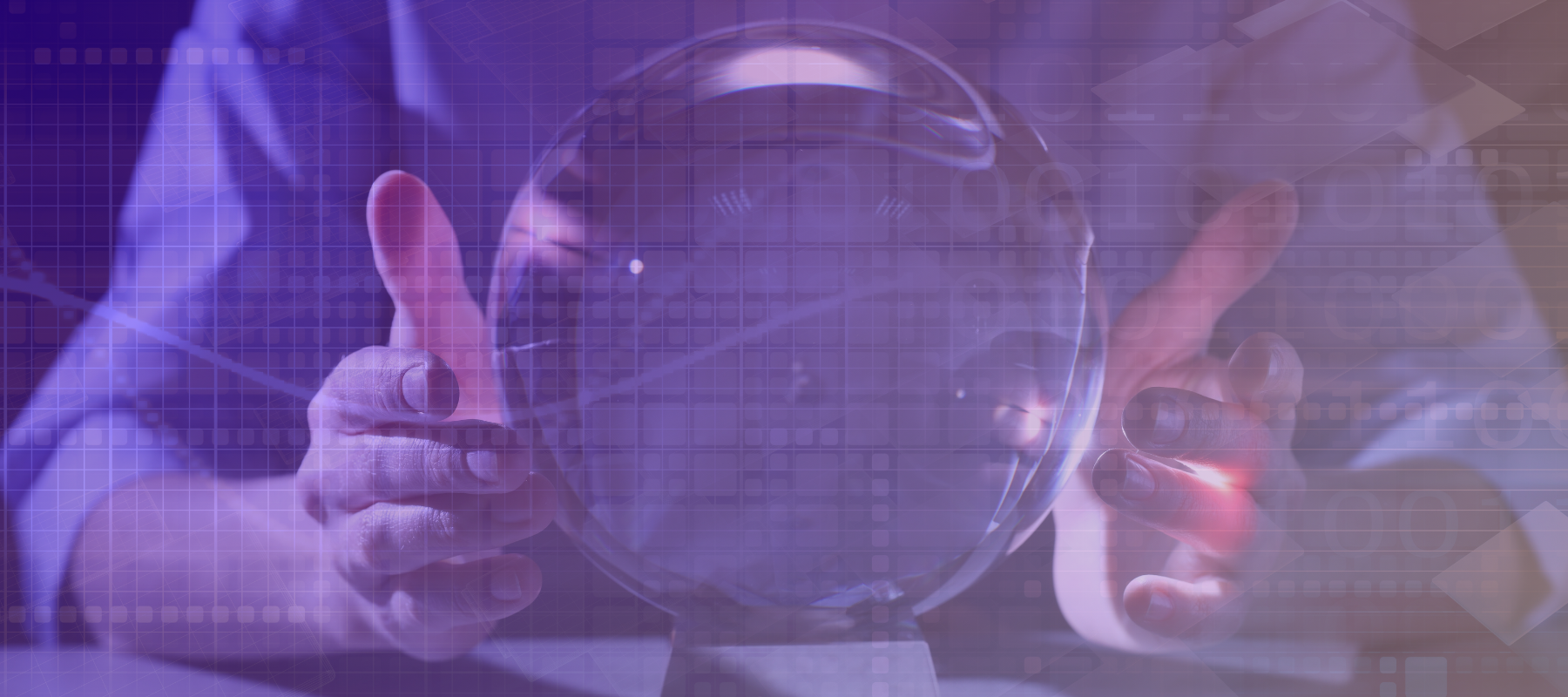
Imagine planning a road trip with a 50-year-old map — sounds risky, right?
Business leaders face a similar challenge when relying on outdated labor market data and analysis to build their future-focused workforce strategies. Real-time, accurate market intelligence is no longer a luxury — it's become critical for organizations undergoing rapid shifts driven by technology and automation.
Traditional methods of gathering and analyzing labor market intelligence, which can include delayed public datasets and limited surveys, often fail to deliver actionable insights when businesses need them most. Modern predictive artificial intelligence (AI) and machine learning (ML) technology, however, empower organizations to quickly analyze large amounts of real-time data aggregated from many diverse sources — including online job postings and skill inventories — to make informed workforce decisions.
With predictive AI-powered analysis, organizations can accurately identify current skill gaps, forecast talent needs, and plan workforce strategies for the future. Here's a closer look at predictive AI's transformative potential and how it equips leaders with the insights needed to face the future head-on.

Common Analysis Challenges for Labor Market Data
Accurate, timely, and comprehensive labor market data is essential for informed decisions on workforce planning, educational policies, and macroeconomic strategies. Significant limitations in data collection or analysis, however, can get in the way of impactful decision-making.
Here are a few common challenges that limit the power of labor market intelligence.
Delayed, outdated information
Would you make business decisions today based on last year's news or data? Probably not. Yet public data often comes out months, or even years, after it's collected. By the time it's available, economic realities have likely shifted.
In the U.K., for example, a two-year delay in updating official labor market statistics has impacted decisions by the Bank of England and the government, depriving policymakers of reliable data and potentially leading to misinformed economic decisions.
And in a world where AI and automation transform jobs overnight, delayed data means missed opportunities to retrain workers or secure in-demand talent.
Limited amounts of data
When you think of labor market statistics, you might expect a broad and accurate snapshot of the current talent landscape, right? Datasets built on limited surveys, however, only tell part of the story. Low response rates and small sample sizes reduce the reliability of this information.
Such discrepancies prevent policymakers from developing effective strategies, leave businesses uncertain about workforce planning and undermine public trust in official statistics, highlighting the need for consistent and modernized data collection methods.
Lack of granularity, depth
As much as governments and organizations talk about sustainability, net-zero emissions goals, and green jobs, most companies don’t know how to take meaningful steps to achieve these goals. Yet the green transition is causing one of the biggest shifts in today's labor market, promising millions of new jobs.
So where exactly will these jobs emerge? And how do we prepare the workforce for them?
The challenge is in the data — more specifically, the lack of depth in the data. Without granularity, it's difficult to pinpoint opportunities in emerging sectors or even anticipate demand. For example, while renewable energy is projected to create more than 10 million jobs globally by 2030, according to a 2024 Staffing Industry Analysts report, governments and industries risk falling behind without the insights to allocate resources effectively. This gap could hinder the green transition, leaving critical sectors under-resourced and workers unprepared.
Insufficient trend analysis
More than half of workers worldwide are expected to need significant reskilling or upskilling by 2030 to keep up with the demands of technological advancements, according to the World Economic Forum. The challenge, however, goes beyond broad reskilling initiatives — employers must also identify the specific skills needed to keep their organizations competitive.
Simply copying trendy phrases such as “data accuracy,” “attention to detail,” or “data validation and quality control” from job post to job post, however, isn't an effective strategy for closing skill gaps. The scope of your job posting must also make sense for your business goals and objectives, too.
Not all industries need the same skills or talent pools to move ahead. Each industry — even each company — faces unique challenges and opportunities that demand tailored workforce strategies. Without a clear understanding of these specific needs, companies risk ineffective workforce planning, delayed innovation, and falling behind in fast-growing markets.
How Predictive AI Transforms Data
Predictive AI is revolutionizing how organizations and industries use data.
Predictive artificial intelligence involves using statistical analysis and machine learning to identify patterns, anticipate behaviors and forecast upcoming events. Organizations use predictive AI to predict potential future outcomes, causation, risk exposure and more, according to IBM's definition.
Depending on the data being analyzed, predictive AI can have a tremendous impact on different roles, functions, and parts of the business. In the airline industry, for example, predictive analytics can help manage air traffic routing to reduce human error or proactively identify and resolve maintenance issues to minimize downtime.
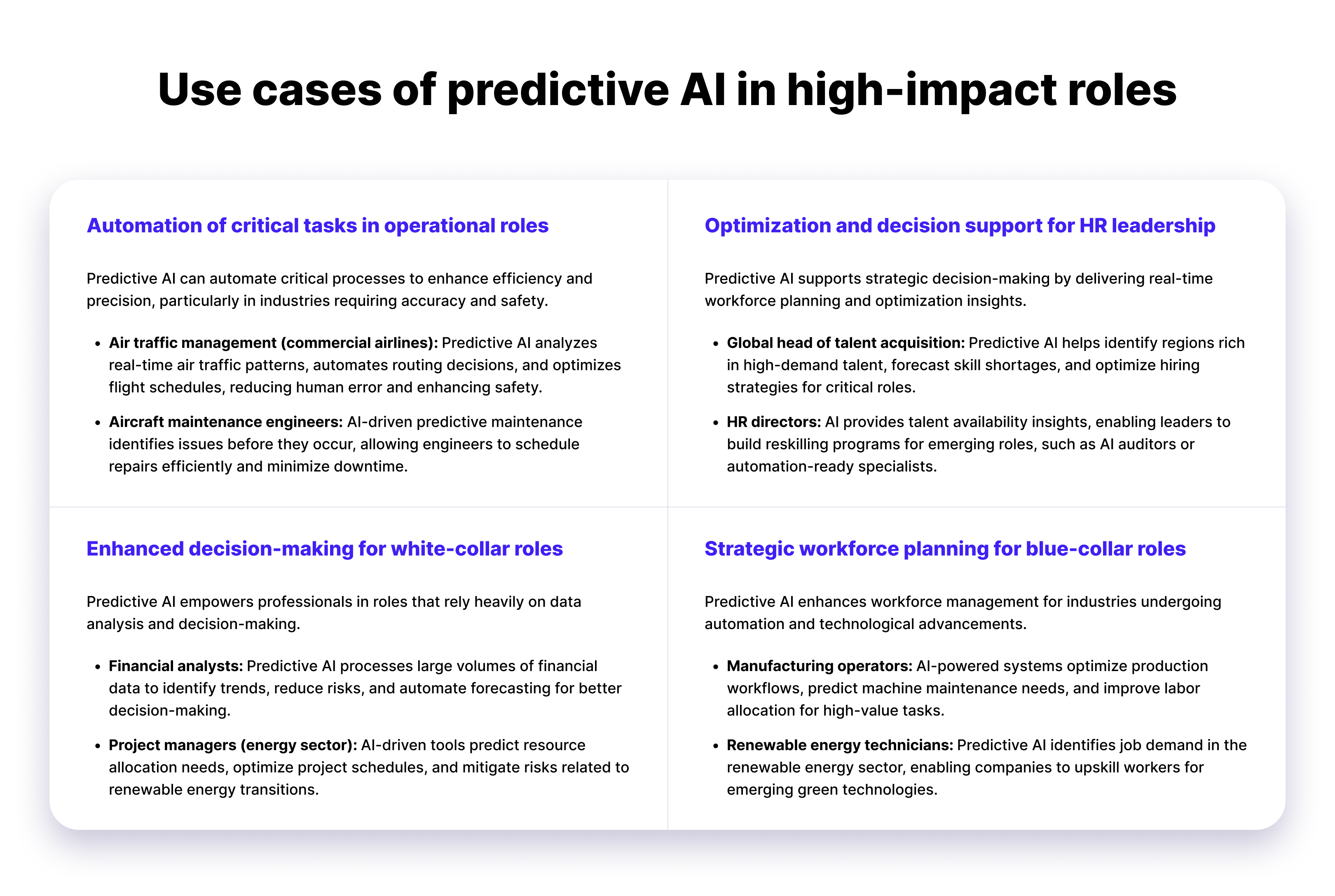
For talent acquisition and HR teams, using predictive analytics helps inform build-borrow-buy decisions on talent. By processing real-time labor market data into actionable insights on skill trends and workforce impact, organizations are able to make informed, agile decisions. This data-driven approach helps bridge talent gaps and achieve transformation goals faster and more effectively.
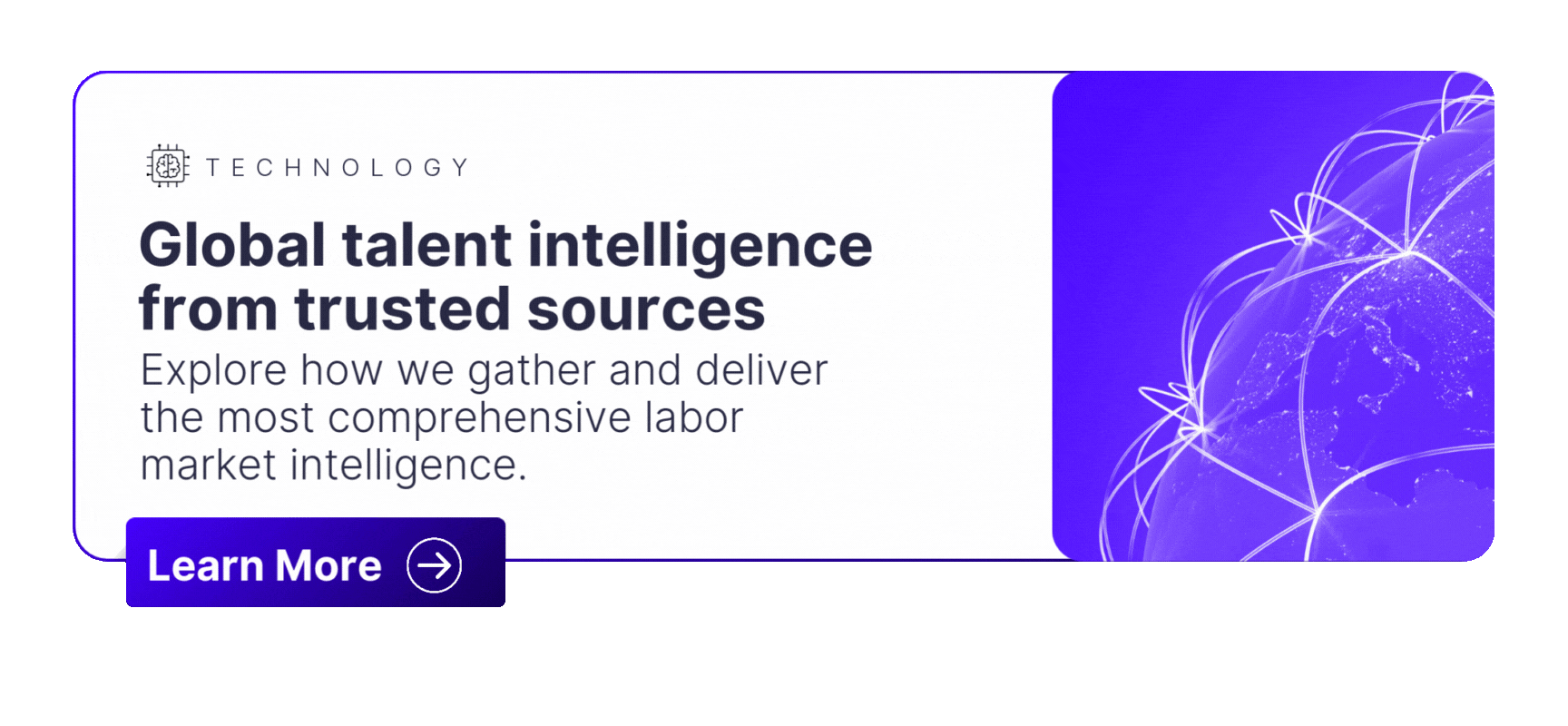
5 Ways Predictive AI Can Boost HR Initiatives
By bridging data gaps, predictive AI opens up new opportunities for HR professionals to implement strategic initiatives.
But it all starts with having the right data and tools to make the most of predictive analysis.
TalentNeuron's predictive AI-powered solutions, for example, draw from the industry's most comprehensive global labor market data, standardizing nearly 3 trillion quality data points gathered from more than 28,000 diverse sources, including government reports, job boards, candidate profiles, and proprietary databases.
These insights empower organizations to:
- Identify necessary skills for workforce transformation and prioritize upskilling and reskilling strategies for critical talent segments.
- Evaluate talent availability and location flexibility using comprehensive global data.
- Streamline recruitment processes by automating repetitive tasks, enabling HR teams to focus on employer branding and personalized candidate experiences.
- Pinpoint regions rich in automation-ready talent to support effective recruitment and workforce planning.
Here's a closer look at how TalentNeuron market intelligence can help navigate a few key workforce decisions.
Strategic workforce planning
Strategic workforce planning (SWP) is a necessity for organizations looking to thrive in the face of rapid technological advancements and competitive pressures. HR and business leaders can build a strong foundation for addressing key workforce challenges by using AI-powered data analysis to:
- Anticipate job role evolution to forecast changes driven by AI and automation to align talent strategies
- Eliminate inefficiencies to replace manual spreadsheet processes with integrated, purpose-built SWP tools.
- Address talent gaps to upskill surplus talent and designing training programs to close skills gaps.
- Optimize hiring to manage internal talent insights to reduce costs and improve hiring success rates.
- Gain competitive advantage by using location analysis and benchmarking to outpace competitors.
JM Family Enterprises, for example, used TalentNeuron analytics to identify emerging skills gaps and implement upskilling programs, increasing readiness for technological advancements and competitive pressures. This success demonstrates how organizations can align talent development strategies with future market needs.
Identifying emerging skill gaps
TalentNeuron's analytics provide organizations with foresight into skills trends, enabling proactive workforce planning and skill gap analysis. By integrating external labor market intelligence with internal skill inventories, HR and business leaders can:
- Prioritize skills essential for digital transformation.
- Analyze competitors' hiring patterns for deeper market insights.
- Predict future skills requirements to achieve transformation goals.
Qualcomm used TalentNeuron analytics to prioritize critical skills for digital transformation, allowing the company to remain competitive by addressing talent shortages before they become critical.
Streamlining recruitment in strategic locations
Did you know that the average cost of a new hire is estimated at $4,700, though employers say that number can go as high as four times the position’s salary, according to the Society for Human Resources Management (SHRM)?
TalentNeuron insights help organizations avoid costly hiring mistakes and high-impact turnover by streamlining talent acquisition and hiring processes. Teams can leverage this to:
- Explore hiring opportunities in different locations with a higher supply of candidates and lower compensation.
- Recommend alternative job titles based on prevailing titles in the market to attract more candidates.
- Provide insight into competitors’ hiring activity for similar roles, which may inform urgency to fill and compensation decisions.
Companies can significantly reduce costly mistakes and drive employee retention by accurately assessing candidates' fit and predicting their skill match to certain roles. They can also assess the automation readiness of certain roles and tasks.
Southwest Airlines, for instance, tapped into TalentNeuron’s automation-ready talent insights to streamline recruitment processes and implement region-specific hiring strategies, ensuring operational efficiency.
Following critical skill and job evolutions
TalentNeuron's comprehensive data includes an extensive collection of jobs, skills, and training resources. This feature facilitates:
- Job standardization that clearly communicates job roles and responsibilities, improving HR processes like candidate assessments and internal mobility
- Skills taxonomy development in a structured framework that enables accurate job descriptions and targeted upskilling programs
- Real-time labor market insights that help optimize recruitment pipelines and identify cost-effective hiring strategies
Deutsche Telekom used TalentNeuron to develop a structured skills taxonomy that not only optimized internal mobility but also increased the accuracy of job descriptions, enabling targeted upskilling programs.
Create competitive employer value propositions
Organizations' internal input, values, and objectives mainly drive employee value propositions (EVPs), but labor market data and insights give them true direction.
- Market trends reveal personas' preferred benefits and perks and the competition for talent across roles, locations, and industries
- Competitive benchmarking of other EVPs and brands lets organizations explore new ways to differentiate themselves in crowded labor markets
- Employer branding gives visibility into how an EVP is perceived by employees and the market, giving organizations a chance to improve offerings and messaging.
Jabil, for example, used TalentNeuron market trends and EVP benchmarking data to stand out in crowded job markets by aligning its employer brand with both employee preferences and competitive standards. This solution ultimately helped them attract better specialists and talent by creating a win-win for the company and future employees.
